Cambridge Healthtech Institute's Inaugural
Predicting Developability and Optimization Using Machine Learning
Accelerating the Development of Complex Biotherapeutics
January 15 - 16, 2025 ALL TIMES PST
Wednesday, January 15
NOVEL PREDICTIVE AND GENERATIVE APPROACHES FOR SOLVING REAL-WORLD ENGINEERING CHALLENGES
Antibody Optimization Combining Structure-Based Approaches and Deep Learning
Alan Cheng, PhD, Senior Director, Modeling and Informatics, Merck Research Labs
In the discovery of antibody therapeutics, experimental molecular biology and biophysical methods are powerful approaches that can be combined with computational approaches to accelerate identification of better molecules. We share case studies illustrating how the close integration of experimental methods and deep learning can identify new leads and how structure-based and deep learning approaches can be used to optimize molecules for developability properties.
A Clinically Validated AI-Driven Platform for Designing Smart and Dynamic Antibody Therapeutics
Reshef Shilon, Head of AI, Biolojic Design
Although antibody drugs have great therapeutic benefits, most function primarily as inert antagonists. They don't fulfill the potential of proteins, which are dynamic machines that can sense changes in the environment and respond to them. Biolojic Design's clinically validated AI-driven platform designs dynamic antibodies that are programmed to respond to changes in the environment The first such antibody is in phase 2. I will describe Biolojic’s platform, which experimentally generates data for AI models. I will focus on the computational aspects, which aim to optimize, simultaneously, multi-antibody properties, which in turn allow the design of smart and dynamic antibodies.
2:35 pmRefreshment Break in the Exhibit Hall with Poster Viewing
Delivering Better Clinical Candidates, Faster: Practical Impacts of Machine Learning on Biologics Preclinical Pipeline
Andrew B. Waight, PhD, Senior Director, Machine Learning, Discovery Biologics & Protein Sciences, Merck Research Labs
AI/ML applications to the field of protein engineering have recently generated immense enthusiasm. In addition to protein folding and de novo design, statistical and deep learning technologies are revolutionizing many aspects of the biologics therapeutic discovery. We cover some of the real-world use cases for AI/ML applications—from hit identification to lead optimization stages of discovery biologics—that are facilitating the computational identification of high-quality preclinical candidates and improving efficiency.
Data Collection Strategies & AI-Ready Discovery Packages
M. Frank Erasmus, PhD, Head, Bioinformatics, Specifica, an IQVIA business
Exploring the bilateral importance of leveraging strategic data collection and intelligent experimental design to enhance AI capabilities in antibody development. Discussing the utilization of evolutionary intelligence to refine and optimize affinity maturation strategies, leading to the discovery of antibodies with superior binding properties and therapeutic potential.
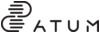
Sridhar Govindarajan, Co Founder & CTO, IT, ATUM
ATUM’s antibody platform combines ML/AI with production quality expression systems and robust analytics. Antibodies are designed in silico, assisted by AI and knowledge base, synthesized at scale in commercially relevant platforms, and are characterized for functionality and developability features simultaneously. ML models built on these datasets which are built specifically to “learn”, are highly predictive and generate new designs for high-specificity antibodies with developability properties for process development, scale-up, and manufacturing.
Generative and Predictive Machine Learning for Iterative Multi-Objective Therapeutic Antibody Optimization
Hunter Elliott, PhD, Senior Director, Machine Learning, BigHat Biosciences
The path from discovered binder to developable modern antibody therapeutics represents a complex protein engineering challenge. At BigHat we frame this as a multi-objective Bayesian optimization problem, with machine learning models in-the-loop with a high-throughput wet lab on a weekly build-test-train cycle. We present several case studies of novel predictive and generative ML methods deployed on this platform to solve real-world antibody therapeutic engineering challenges.
This Town Hall discussion offers delegates the opportunity to participate in an interactive discussion on important themes that were explored during the conference. The co-hosts will facilitate the conversation, and all are welcome to participate, share views and best practices and ask questions of colleagues.
Applying AI to Improve Manufacturability and Developability of Multispecific Biologics
Jeffrey J. Gray, PhD, Professor & Research Mentor & Outreach Advisor, Chemical & Biomolecular Engineering, Johns Hopkins University
- Improving humanization and predicting immunogenicity
- Reducing off-target binding
- Predicting aggregation, viscosity, and excipient formulation
- Combining targets for improved efficacy
Wednesday Night Meet-Up
CANCELLED: What Needs to be Done to Make a Pipeline of Mini-Binders More Developable?
Monica L. Fernandez-Quintero, PhD, Staff Scientist, General Inorganic & Theoretical Chemistry, Scripps Research Institute
Wing Ki Wong, PhD, Senior Scientist, Pharmaceutical Research and Development, Large Molecule Research, Roche Diagnostics GmbH
Network, Inspire Others and Connect
- Get to know fellow peers and colleagues
- Make connections and network with other institutions
- Inspire others and be inspired!
We will meet outside of the exhibit hall then transition to the lounge area
Thursday, January 16
7:45 amRegistration and Morning Coffee
PLENARY KEYNOTE SESSION
Transforming Therapeutic Protein Engineering
Marissa Mock, PhD, Executive Director Research, Amgen Inc.
Generative biology is an emerging discipline that integrates artificial intelligence (AI) and machine learning (ML) with advanced life science technologies. The application of generative biology to protein engineering is accelerating the discovery and design of complex proteins with therapeutic potential—and, maximizing the benefits of these novel technologies will require seamless integration of both wet- and dry-laboratory technologies.
8:50 amSession Break
INTEGRATING AI/ML FOR ENHANCED ANTIBODY SELECTION AND DEVELOPABILITY
Generative AI-Guided Design of Vaccine Immunogens
Reda Rawi, PhD, Staff Scientist and Co-Head, Structural Bioinformatics Core, NIH NIAID
Structure-based vaccine design campaigns that aim to stabilize full-length proteins will not succeed when the virus is evading immune response by sequence diversity. In this work, we capitalized on the recent major advanced that have been achieved in protein design using generative AI tools. We in silico designed de novo proteins that scaffold sequence-conserved epitope regions of the antigens of interest. Next, we incorporated the in silico designed mini proteins onto self-assembling nanoparticles and performed pre-clinical animal immunization studies eliciting immune responses.
ML Optimization of Candidate Antibody Yields Highly Diverse Sub-Nanomolar Affinity Libraries
Lin Li, PhD, Senior Staff Member, Lincoln Laboratory, Massachusetts Institute of Technology
The design and discovery of early-stage antibody therapeutics is time- and cost-intensive. I will present an end-to-end machine learning-driven single-chain variable fragments (scFv) design framework that uniquely combines large language models, Bayesian optimization, and high-throughput experimentation. The method enables rapid and cost-effective design of thousands of scFvs across all complementary determining regions. The designed antibodies exhibit strong binding affinities, at high levels of diversity, to a given antigen.
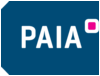
Sebastian Giehring, PAIA Biotech GmbH
In this presentation, we will critically evaluate the current methods for generating early developability data, identify existing shortcomings, and present innovative high-throughput assays utilizing the plate-based PAIA technology. This method is designed to deliver reliable and unambiguous biophysical developability data, serving as an efficient replacement for time-intensive and laborious HPLC and ELISA methods.
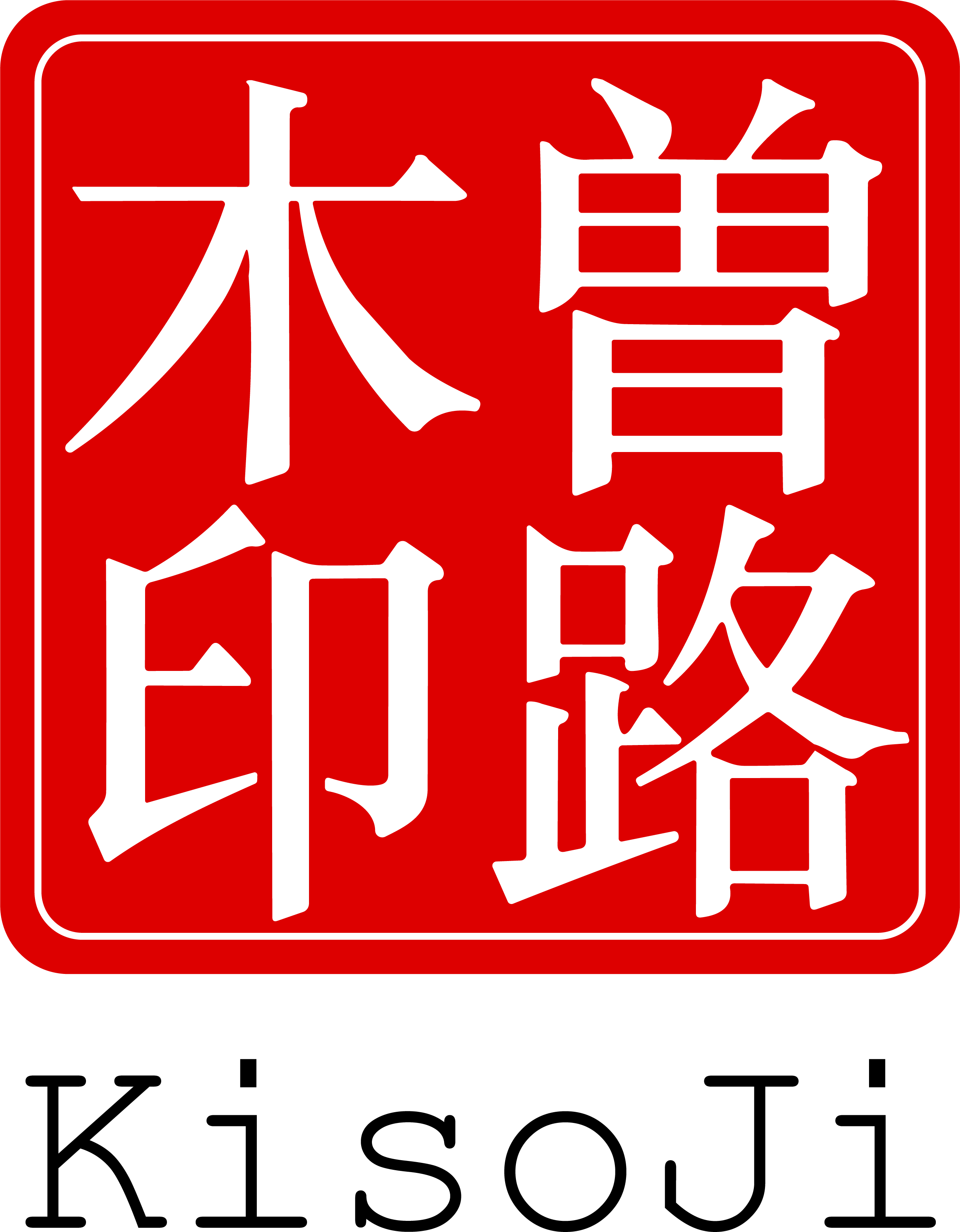
Morteza Babaie, Senior AI Scientist, Kisoji Biotechnology Inc
The KisoSeek™ platform leverages AI-driven paratope mapping to accelerate therapeutic antibody discovery. Integrating autoencoders, structural/sequence data and large language models to visualize antibody repertoires in multidimensional maps, enabling functional clustering. Built on KisoMouse(R) HCAb immunization libraries, this approach improves the exploration of diversity through various sampling strategies, including probability-based and targeted selections. KisoSeek™ supports VHH and VH-VL antibodies, driving efficient and versatile candidate selection for therapeutic development.
10:30 amCoffee Break in the Exhibit Hall with Poster Viewing
Efficient Evolution of Antibodies from General Protein Language Models
Varun Shanker, MD-PhD Student, Biophysics, Stanford University
This talk will cover how large language models of protein sequences and structures can learn evolutionary rules that help guide the artificial evolution of human antibodies. We will cover how algorithms known as protein language models can guide the affinity maturation of antibodies against diverse antigens using sequence information alone. Next, we will cover how multimodal language models can further improve the ability to guide antibody evolution completely unsupervised by incorporating information about the protein's structure, which we use to make clinical antibodies more potent and resilient against viral escape variants.
TABLE 8: AI/ML-Driven Design of Conditionally Active Molecules
Hunter Elliott, PhD, Senior Director, Machine Learning, BigHat Biosciences
- What is the therapeutic potential of conditional activity and how do we best balance this against increased complexity and risk?
- What challenges are unique to ML-driven design of conditional molecules?
- How does the optimal ML toolkit vary between conditional and unconditional design?
- How best can we overcome challenges in data acquisition and availability?
- What are the currently tractable forms of conditional activity and what can we envision for the future?
12:30 pmSession Break
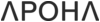
Shamit Shrivastava, CoFounder & CEO, Apoha
The Liquid Brain®, Apoha’s novel physical ecosystem, introduces state diagrams: a new class of time-resolved, high-dimensional biophysical data that grounds AI/ML protein models in physical reality. While existing data often fails to capture the dynamic, real-world behaviour of molecules, the Liquid Brain® seamlessly bridges this gap via physics of excitable substrates. Designed with an AI-first mindset, the Liquid Brain® directly interacts with samples to generate predictive insights. Using just 10μg of antibodies, it performs single-shot classification of developability risks, validated against 12 biophysical assays across 135 clinical-stage antibodies. In a customer study, it identified outliers in 70 VHH-Fc fragments with >80% accuracy via simple unsupervised algorithms and without any training.
Our dataset of risk scores for 135 clinical stage antibodies is now available on Hugging Face. Join us to discover how the Liquid Brain® can empower you with ML ready state diagram embeddings!
1:10 pmIce Cream & Cookie Break in the Exhibit Hall with Last Chance for Poster Viewing
INTEGRATING AI/ML FOR ENHANCED ANTIBODY SELECTION AND DEVELOPABILITY
Towards Enhancement of Antibody Thermostability and Affinity by Computational Design in the Absence of Antigen
Gilad Kaplan, PhD, Director, Biologics Engineering, AstraZeneca
DeepAb, a deep learning model for predicting antibody Fv structure directly from sequence, was used in conjunction with experimental deep mutational scanning (DMS) enrichment data to design 200 potentially optimized variants of an anti-hen egg lysozyme (HEL) antibody. We discuss the improvement rate of the designed clones for affinity, thermostability, and developability—and what the results would have been without using experimental DMS data to guide the design process.
Towards Optimal Clone Selection: Enhancing Antibody Discovery with AI/ML Approaches
Adrian Carr, PhD, Associate Director, Data Science, AI Innovation—Antibodies, Large Molecules Research (LMR), Sanofi
Optimal clone selection strikes a balance between selecting desirable antibody properties and diversity, aiming to maximize the range of targeted epitopes while minimizing redundancy. At Sanofi, we are exploring in silico screening, leveraging AI/ML methods to enhance success rates and accelerate the transition from discovery to lead. In parallel, generative AI can be used to augment natural and experimental repertoires, eliminating undesirable traits while preserving desirable characteristics.
AI DRIVEN OPTIMIZATION OF ANTIBODY PROPERTIES
M. Frank Erasmus, PhD, Head, Bioinformatics, Specifica, an IQVIA business
- Where has there been the most benefit to cost or time savings?
- Are we noticing developability tools showing improvements in preclinical studies?
- Are we noticing reduction in immunogenicity or timeline?
- Where does in silico modeling allow us to remove experimental efforts and use computational modeling to replace?
- Have we seen benefits to the implementation of Federated Learning?
- Are we seeing benefits to optimization and developability?
- Where do we see the most promising high throughput developability screening capabilities to facilitate AI model training?
3:35 pmClose of BioLogic Summit